Pricing and Competition for Generative AI – Rafid Mahmood #NeurIPS2024
If you’re interested in the recent NeurIPS2024 event , then you’ll benefit from this website: NeurIPS 2024 Posters, interactive visualization. I found this Pricing and Competition for Generative AI paper by choosing “Filter by manually assigned topic” by “Social Aspects”.
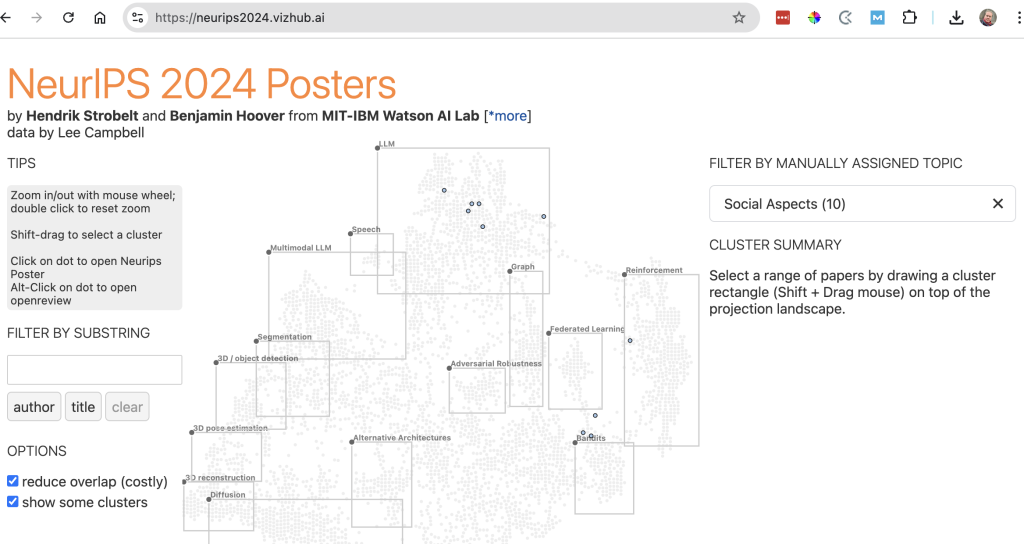
I wanted to “surface” this paper because — although Dear Reader for this paper is sell-side AI model companies — I think it’s important for buy-side AI practitioners to grok the concepts and understand how the sellers approach the market.
You can get the paper from Arxiv: Pricing and Competition for Generative AI – Rafid Mahmood – NVIDIA &University of Ottawa
A simplified synopsis for pricing and competition for Generative AI models.
As usual with all papers, this is an information dense paper, and I wanted to “loosen it up” a bit and make more understandable for the more casual AI practitioner.
1. Generative AI Models vs. Traditional Machine Learning
Generative AI models are different from traditional machine learning (ML) models because:
One Model, Many Uses
You can use one generative model for various tasks without needing to train new models for each task.
User Interaction
People use these models by giving them instructions in everyday language.
Success Measure
The main way to judge if the model is good is whether users like its outputs or not (yes or no).
2. Releasing and Pricing Generative AI:
The paper looks at how companies should introduce and set prices for their generative AI software:
Model Comparison
They compare two models to see which one gives users more value for money for a specific task.
Pricing Strategy
They treat the pricing as a competitive game where:
- Two companies release their models one after another.
- Users then choose which model they prefer for each task.
- Pricing becomes tricky because companies need to decide which tasks to focus on for cost-effectiveness, potentially losing out on revenue from other tasks.
3. Key Findings
Market Timing
The company releasing its model later has an advantage because they can adjust their prices knowing what the first company did. They can always make sure their model is cost-effective for at least one task.
The first company to release must set prices in a way that might encourage the second company to charge more, which could help the first company earn more.
Task Similarity
In summary, the study discusses how generative AI can be priced and released in a market where timing and understanding of competitor actions play significant roles in success.
What’s in the paper?
Here’s an outline for an explanatory document based on the paper “Pricing and Competition for Generative AI” by Rafid Mahmood:
1. Introduction
- Briefly discuss how generative AI models differ from traditional machine learning by their ability to handle multiple tasks through natural language prompts.
- Explain that the paper explores pricing strategies for generative AI in a competitive market.
2. Key Characteristics of Generative AI Models
- Multi-Task Capability: One model can perform various tasks without retraining.
- User Interaction: Interaction via natural language prompts, allowing for iterative use until satisfaction.
- Evaluation: Focus on user satisfaction as a binary measure rather than traditional accuracy metrics.
3. Problem Statement
This is interesting if *also* consider this from the buy-side. How can this help you, as a buyer/admin/practitioner of AI?
- Pricing Challenge: Discuss the complexity of pricing a generative AI model which can be used for many tasks, each with different user demands.
- Market Dynamics: Introduce the competitive environment where multiple AI models exist, affecting pricing strategies.
4. Model Framework
- Tasks: Define what constitutes a task in the context of generative AI, emphasizing the binary evaluation of task completion.
- Model Definition: Describe how models are characterized by price, performance across tasks, and user interaction patterns.
- Demand Functions: Explain how user demand for using a model for a specific task decreases with price.
5. User Preference and Cost-Effectiveness
- Preference Model: Detail how users choose between models based on the price-performance ratio, considering the expected number of prompt rounds.
- Multi-Round Interaction: Discuss the assumption of continued use until satisfaction is achieved.
6. Pricing Strategy in a Competitive Environment
- Game Theory Context: Explain the scenario as a game between two firms:
- First-to-market (Firm A): Sets price without knowing competitor’s move.
- Latecomer (Firm B): Benefits from observing Firm A’s price before setting their own.
- Optimization Problem: Describe how pricing becomes a piecewise continuous problem where firms focus on certain tasks for cost-effectiveness.
7. Key Findings
- Competitive Advantage: Firm B can always secure revenue on at least one task due to having more information.
- First-Mover Challenges: Firm A must strategically price to influence Firm B’s high pricing, or risk becoming cost-ineffective.
- Task Similarity: If tasks are too similar, the first-to-market model might not be cost-effective regardless of pricing.
8. Implications for AI Developers
- Strategic Deployment: Advise on when to deploy a model, considering performance differentiation across tasks.
- Market Information: Emphasize the importance of understanding competitor models’ performance and market demands.
9. Limitations
- Assumptions: Highlight assumptions like known demand functions and model performance.
- Static Market: Discuss how the model assumes a static market, not accounting for dynamic improvements from data collection.
10. Societal Impact
- Technology Access: Discuss how pricing can influence the accessibility of AI technology.
- Ethical Considerations: Mention potential societal implications like democratization versus monopolization of AI capabilities.
11. Conclusion
- Summary: Recap the key insights on pricing generative AI models.
- Future Research: Suggest areas for further study, like dynamic pricing models or considering development costs.